Our life depends on the weather. At any point in the UK, according to one studyA third of the country reported the weather in the past hour, reflecting the importance of weather in daily life. Among weather phenomena, rain is particularly important because of its influence on our daily decisions. Should I take an umbrella? How should we drive vehicles exposed to heavy rain? What safety measures do we take for outdoor events? Will there be a flood? Our latest research And a modern model develops science Precipitation forecast now, which is a forecast of rain (and other precipitation phenomena) within the next 1-2 hours. in paper Written in collaboration with the Met Office and published in Nature, we get straight to this important matter Big challenge in forecasting the weather. This collaboration between environmental science and AI focuses on value for decision makers, opens new avenues for nowcasting rainfall, and points to opportunities for AI in supporting our response to decision-making challenges in an environment subject to constant change.
Short term weather forecasts
Throughout history, weather forecasts have held a place of importance to our communities and countries. Medieval meteorologists began using the stars to make predictions. Slowly, tables recording the seasons and rain patterns began to be kept. Centuries later, Louis Fry imagined a “forecast factory” that would use math and physical equations of the atmosphere to predict global weather. In this evolving weather forecasting book, we now add a story about the role of machine learning in forecasting.
Today’s weather forecasts are driven by powerful Numerical Weather Prediction (NWP) systems. By solving physical equations, NWP provides basic planet-wide forecasts several days in advance. However, they struggle to generate high-accuracy predictions for short time periods of less than two hours. Nowcast fills the performance gap in this crucial time period.
Nowcasting is essential for sectors such as water management, agriculture, aviation, contingency planning, and external events. Advances in weather sensing have made high-resolution radar data — which measures the amount of precipitation at ground level — available at a high frequency (eg, every 5 minutes with 1 km resolution). This combination of the critical area where current methods struggle and the availability of high-quality data provides the opportunity for machine learning to make its contributions to nowcasting.
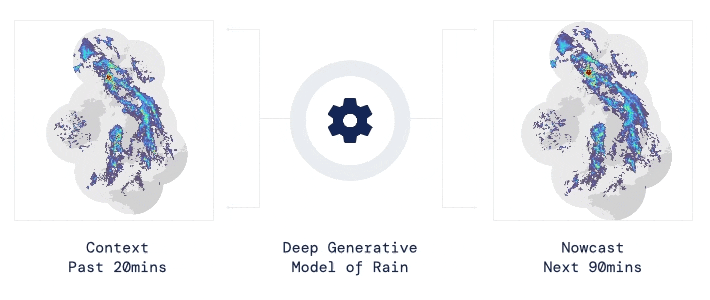
Generative models of real-time broadcasting
We focus on realtime rain forecasting: forecasts up to two hours in advance that capture the amount, timing, and location of rain. We use an approach known as generative modeling to make detailed and reasonable predictions of future radar based on past radar. Conceptually, this is a problem with generating radar movies. Using these methods, we can accurately capture large-scale events, while generating several alternative rain scenarios (known as ensemble forecasts), allowing exploration of rainfall uncertainty. We used radar data from both the United Kingdom and the United States in the results of our study.
We were particularly interested in the ability of these models to make predictions about moderate to heavy rain events, events that have the greatest impact on people and the economy, and show statistically significant improvements in these systems compared to competing methods. Importantly, we conducted a cognitive task assessment with more than 50 meteorologists at the Met Office, the UK’s national meteorological service, who rated our new approach as their first choice in 89% of cases when compared to widely used telecast methodsdemonstrating the ability of our approach to provide insights to real-world decision makers.
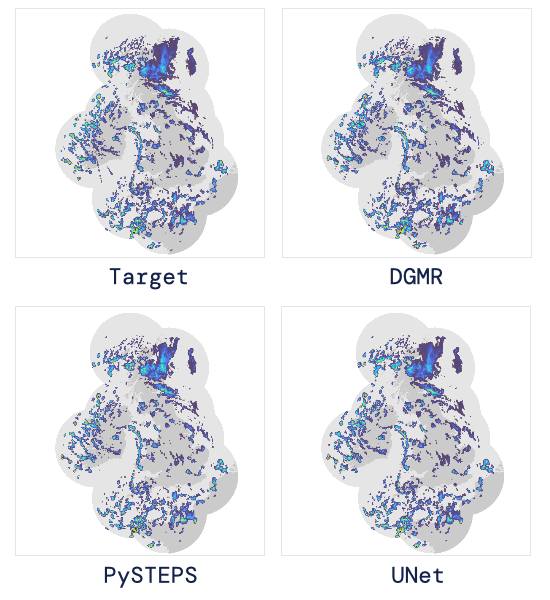
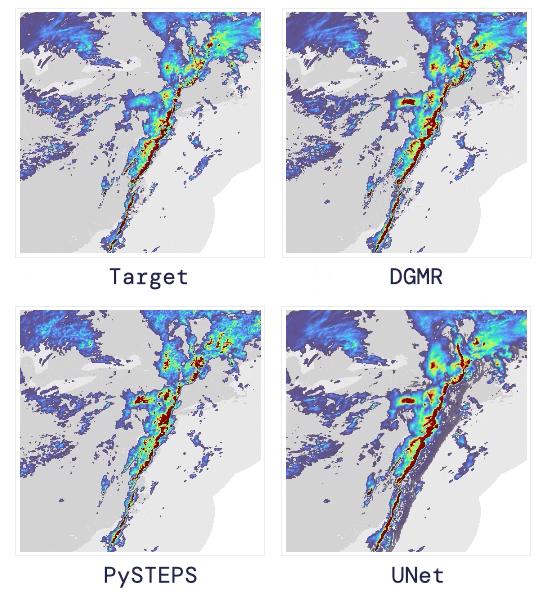
What then
Using statistical, economic, and cognitive analyses, we have been able to demonstrate a novel, competitive approach to estimating precipitation now from radar. No method is without limitations, and more work is needed to improve long-range prediction accuracy and accuracy for rare, intense events. Future work will require us to develop additional methods for performance evaluation, and to further tailor these methods to specific real-world applications.
We believe this is an exciting area of research and hope that our paper will serve as a basis for new work by providing data and validation methods that make it possible to provide competitive validation and operational benefit. We also hope that this collaboration with the Met Office will promote greater integration of machine learning and environmental science, better supporting decision-making in our changing climate.
Read the article Instant Precipitation Prediction Using Generative Deep Radar Models in the September 30, 2021 issue of Nature, which contains an extensive discussion of the model, data, and validation approach. You can also explore the data we used for training and find a pre-trained model for the UK via GitHub.